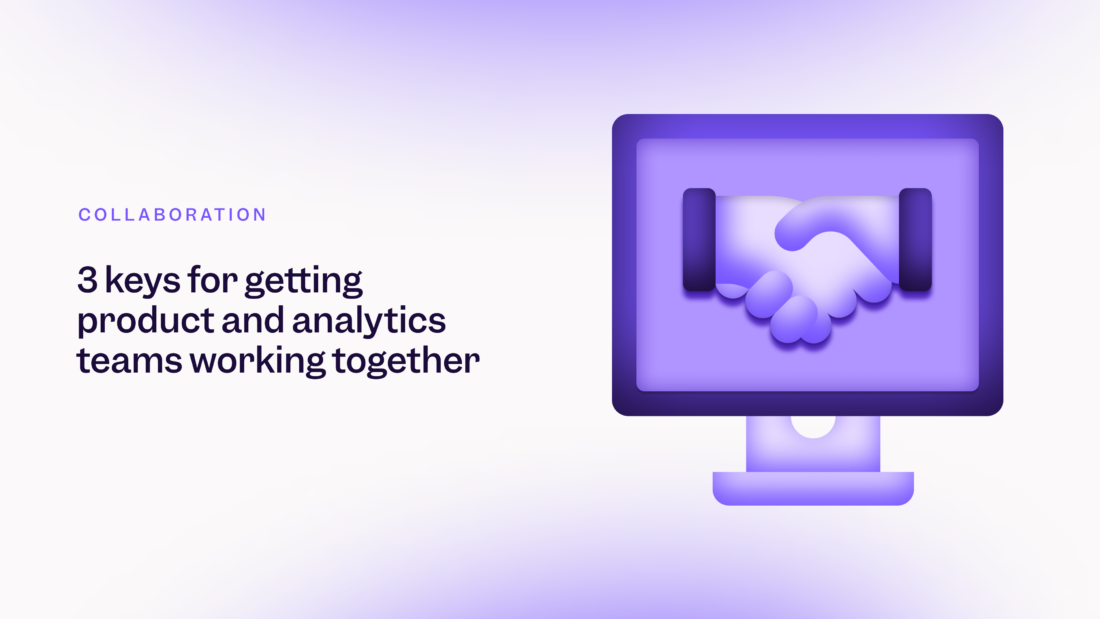
How to get product and analytics teams at large companies working together in lockstep
It's all about data democratization, self-serve analytics, and more inclusive product and feature launch sprints.

I recently went for a hike with my two daughters in the hills that surround our home. Along the way, one of my daughters suggested that we walk in one line and coordinate our steps.
She knew this was no simple task. Our legs have different lengths, each of us walks at a different pace, and there were changes in terrain so we couldn’t only focus on coordinating our feet as we went along.
This experience reminded me a lot of my work as an analytics leader at AT&T. Hear me out: In my role, I’m tasked with working stride-for-stride with the product teams across our large company to arm them with the data insights needed to improve their products. Keeping pace and maintaining alignment can be essential for the ultimate direction and success of our core product offerings.
Interestingly, I’ve found the answers for both literally and figuratively walking together as a team to be similar: Don’t rely on hand-holding. It’s easier to go further faster when everyone participating has the knowledge and empowerment to step forward with confidence.
Since you probably came here to read how these principles apply to my day job rather than walks with my daughters, I’ll cut right to it. Below is how I apply the above keys to unlock product team and analytics team collaboration at large companies.
Cracking through silos at big companies
Let me start by setting the stage a little.
If you’ve ever worked at a startup, you know things at companies that size move fast and team members are often asked to pitch in on projects that are technically outside of their scope. This is why startups traditionally need generalists. Large tech companies tend to be the opposite, though: With more teams needed to handle all the work of the different arms of the org simultaneously, there’s more need for specialists.
At large companies, where everyone is heads-down and intensely focused on their area of work, silos can form. And just because teams are regularly interfacing (handing off projects, making information requests, etc.), that doesn’t mean they share enough knowledge of what the other does to actually efficiently “work together.”
Since efficiency should be the goal of any size company, breaking down those silos with shared understanding, empowerment, and continued communication is an important endeavor—especially between teams as integral as product and analytics.
Shared understanding
As a member of the analytics team tasked with deriving insights for product teams, I need to know more than just how to build dashboards and metrics visualizations. Understanding the products themselves is tremendously important, including things like the different user flows, buttons, and various versions across platforms.
All of this context allows us to move faster to deliver richer insights that can identify user trends and inform our recommendations for product development.
The same concept works in reverse. When product teams know enough about how analysis is done—what’s being measured, how it’s being measured, how all of that can be applied to development—they understand the right questions to ask of their data and, in turn, ask of my team. For example, if someone from the product team already knows about all of the user steps (or events) in the product that are being tracked between signup and paid conversion, they can ask a much smarter and more specific question of the analytics team to suss out where user friction is occurring.
Through data democratization, or a dedication to organizing and structuring data in a way that’s easy for people inside and outside the data team to understand, it’s a far smaller task to keep product team members up to speed on how your product analytics operates.
What does data democratization look like? In product analytics, product usage data is captured and analyzed as events. To keep this kind of data organized, or democratized, you should:
- Ensure product user events are labeled properly and comprehensively
- Use event properties or dimensions to keep your number of tracked events from ballooning
- Document thorough event and property descriptions in a data dictionary or glossary
- Create some kind of analytics requirements document (ARD), similar to the product team’s product requirements document (PRD), to spell out how all these events and properties tie into North Star or overall product health metrics
In Mixpanel, my event analytics platform of choice, it’s easy for analytics and data teams and admins to keep events and properties organized and browseable (and properly defined in Lexicon). This makes it possible for product team members, as well as other collaborating teams across the company, to have visibility on what’s being measured in our product and, even perhaps most importantly, learn how to check in on metrics for themselves.
Empowerment
To that last point, data democratization can vastly improve the quality of queries that analytics teams receive, sure, but it’s also a great big step toward another workflow breakthrough: self-serve analytics, or enabling collaborators outside of the data team to run their own reports and get answers to data questions themselves.
Of course, centering your workflow around an analytics platform that offers self-serve querying and report-building features is what makes this possible. Unless, that is, you want to give everyone on your product team SQL lessons and access to IDEs. (I don’t recommend this.)
Instead, a platform like Mixpanel lets anyone with an understanding of the data-tracking plan who can operate a mouse build their own reports and dashboards full of answers to the most common product usage questions, like:
- Have monthly active users (MAUs) increased since we launched that awareness campaign?
- Is anyone using the new feature we spent all of last quarter launching?
- Where in the app are most users dropping off after signing in for the first time?
And self-serve analytics doesn’t have to be limited to low-hanging fruit analysis. Mixpanel, in particular, can go deeper with powerful cohorting and breakdowns that enable next-level insights without a steep learning curve.
At AT&T, it’s been an incredible experience seeing the product managers make decisions based on reports they built. In addition, QA teams are doing analysis and monitoring autonomously, and development groups are finding bugs based on their own anomaly detections in metrics. All of this frees up our analytics team to focus on the important tasks only we can do, like data governance for those self-serve analytics, heavy SQL reports, in-depth analysis using ML tools, running A/B tests, and implementing new tracking plans that keep pace with changes to the product.
Continued communication and collaboration
Once you’re humming on all of the above, the next step is to ensure your workflows adapt as growth and other changes come to your product, and consequently, your product analytics plan. After all, a product that is standing still is the same as a product that is going backward.
The way to get this done is by having analytics, product, and any other collaborating teams locked in on the same development timeline and product roadmap. I’ve created a sprint matrix, from the analytics perspective, that’s worked at organizations I’ve helped lead over the years:
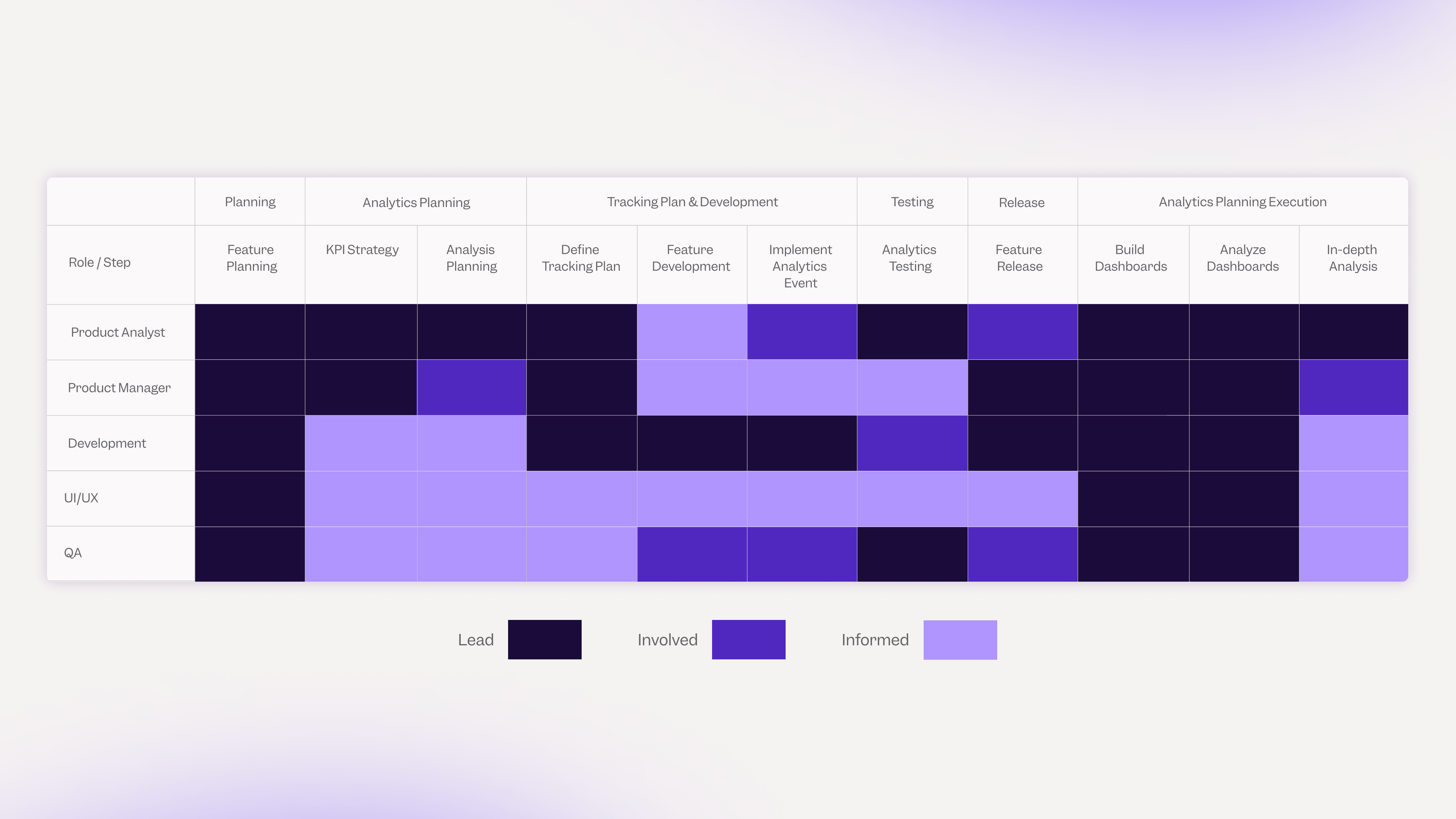
The specific degree of involvement (lead/involved/informed) spelled out above can certainly vary from one feature sprint to another, one product launch to another, or according to different team makeups. But the key is to have a default-to-informed approach for all teams throughout. There are endless numbers of times I’ve run into friction points in roadmap processes, and if you’re asking supporting teams to be reactive (catch up on the information in order to help) rather than proactive (already informed and ready to jump in), your chances of keeping to schedule is greatly improved.
Here’s a bit more about the roles of product team and analytics team members for some of the steps from my sprint matrix:
- While product managers are obvious leads for the Feature Planning stage, each team brings their expertise to the table to help synthesize what a great feature should look like. For analysts, this could be prior product usage information. The process and learnings at this stage will help both product and analytics team members gain the best understanding of the feature that is being developed, which can be applied throughout all following sprint steps.
- In Analytics Planning the product analysts plan the analytical work on new features or products, which are about to enter development with the feature itself and are under their responsibility. It makes sense to divide this step into two parts:
- The KPI Strategy part is where product metrics around a new feature or product are defined or refined. The product team will have input here since they’re just as invested in measuring the overall health of the product as anyone. The Mixpanel Guide to Product Metrics can be used to get some ideas on how to develop measurement strategies.
- In Analysis Planning, the analytics team identifies the analyses that will be needed to connect to the agreed-upon metrics. Which data methods or combination of metrics will get us our answers? Obviously, more questions will arise after we push the feature live, but this planning will help us better reflect to our partners what we are going to analyze, how long it will take, and what data is required.
- The Tracking Plan, led by the analytics team, considers the new feature/product definition and how it will be measured in order to spec out the relevant data events and properties, their timings (clicks, swipe, backend processes, etc.), their formats, the information we will capture from previous events, and more.
- The Feature Release step refers to the moment your new feature goes live. This is an all-hands-on-deck moment, as you want to know as soon as possible if there is a problem with the feature that went overlooked or if there is some kind of existing metric that has dropped sharply since you launched this feature. Alternatively, if the feature works well, that is also something everyone should be aware of (in order to share celebratory beers, of course).
- After the dust has settled, the Analytics Planning Execution period can commence and begin delivering learnings for all teams about the new feature or product. These insights often help us decide what feature to add next, at which point we start a whole new sprint!
It’s a process
If all of the above sounds like a decent amount of extra work, rest assured that the return on investment is far worth it. Using the combination of data democratization, self-serve analytics, and a more inclusive sprint matrix has proven to be a game changer for efficiency between the product teams and the analytics teams at every company I’ve worked.
In fact, if I had a fourth key to suggest, it would be to get started with these practices sooner than later. Trust me, taking on a bit of effort to set up collaboration best practices is far better than putting it off and having one team carry the other for an extended period of time. In the business world, that creates lots of org friction. In the world of fatherhood, it creates a sore back and probably means fewer hikes in the near future.
Happy teamworking!
About Asaf Lev Ari
Asaf is a mission-driven data science and analytics leader with 10+ years of experience both at startups and big companies. Since 2021, Asaf has been managing product analytics teams at the AT&T Israel R&D Center.
Gain insights into how best to convert, engage, and retain your users with Mixpanel’s powerful, self-serve analytics. Try it for free.